Clinical Text Classification with Word Embedding Features vs. Bag-of-Words Features
Document Type
Conference Proceeding
Publication Date
1-22-2019
Journal
Proceedings - 2018 IEEE International Conference on Big Data, Big Data 2018
DOI
10.1109/BigData.2018.8622345
Keywords
clinical text mining; natural language processing; word embedding
Abstract
© 2018 IEEE. Word embedding motivated by deep learning have shown promising results over traditional bag-of-words features for natural language processing. When trained on large text corpora, word embedding methods such as word2vec and doc2vec methods have the advantage of learning from unlabeled data and reduce the dimension of the feature space. In this study, we experimented with word2vec and doc2vec features for a set of clinical text classification tasks and compared the results with using the traditional bag-of-words (BOW) features. The study showed that the word2vec features performed better than the BOW-1-gram features. However, when 2-grams were added to BOW, comparison results were mixed.
APA Citation
Shao, Y., Taylor, S., Marshall, N., Morioka, C., & Zeng-Treitler, Q. (2019). Clinical Text Classification with Word Embedding Features vs. Bag-of-Words Features. Proceedings - 2018 IEEE International Conference on Big Data, Big Data 2018, (). http://dx.doi.org/10.1109/BigData.2018.8622345
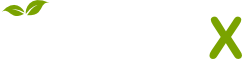
- Citations
- Citation Indexes: 41
- Policy Citations: 1
- Usage
- Abstract Views: 22
- Captures
- Readers: 55