Machine Learning: A Potential Therapeutic Tool to Facilitate Neonatal Therapeutic Decision Making
Document Type
Journal Article
Publication Date
7-1-2024
Journal
Paediatric drugs
Volume
26
Issue
4
DOI
10.1007/s40272-024-00638-6
Abstract
Bacterial infection is one of the major causes of neonatal morbidity and mortality worldwide. Finding rapid and reliable methods for early recognition and diagnosis of bacterial infections and early individualization of antibacterial drug administration are essential to eradicate these infections and prevent serious complications. However, this is often difficult to perform due to non-specific clinical presentations, low accuracy of current diagnostic methods, and limited knowledge of neonatal pharmacokinetics. Although neonatal medicine has been relatively late to embrace the benefits of machine learning (ML), there have been some initial applications of ML for the early prediction of neonatal sepsis and individualization of antibiotics. This article provides a brief introduction to ML and discusses the current state of the art in diagnosing and treating neonatal bacterial infections, gaps, potential uses of ML, and future directions to address the limitations of current studies. Neonatal bacterial infections involve a combination of physiologic development, disease expression, and treatment response outcomes. To address this complex relationship, future models could consider appropriate ML algorithms to capture time series features while integrating influences from the host, microbes, and drugs to optimize antimicrobial drug use in neonates. All models require prospective clinical trials to validate their clinical utility before clinical use.
APA Citation
Tang, Bo-Hao; Li, Qiu-Yue; Liu, Hui-Xin; Zheng, Yi; Wu, Yue-E; van den Anker, John; Hao, Guo-Xiang; and Zhao, Wei, "Machine Learning: A Potential Therapeutic Tool to Facilitate Neonatal Therapeutic Decision Making" (2024). GW Authored Works. Paper 5373.
https://hsrc.himmelfarb.gwu.edu/gwhpubs/5373
Department
Pediatrics
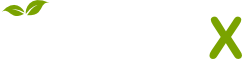
- Citations
- Citation Indexes: 1
- Usage
- Abstract Views: 3
- Captures
- Readers: 6
- Mentions
- News Mentions: 1