Atherosclerosis Imaging Quantitative Computed Tomography (AI-QCT) to guide referral to invasive coronary angiography in the randomized controlled CONSERVE trial
Document Type
Journal Article
Publication Date
2-27-2023
Journal
Clinical cardiology
DOI
10.1002/clc.23995
Keywords
CCTA; artificial Intelligence; atherosclerosis; coronary artery disease; coronary computed tomography; fractional flow reserve; quantitative coronary angiography
Abstract
AIMS: We compared diagnostic performance, costs, and association with major adverse cardiovascular events (MACE) of clinical coronary computed tomography angiography (CCTA) interpretation versus semiautomated approach that use artificial intelligence and machine learning for atherosclerosis imaging-quantitative computed tomography (AI-QCT) for patients being referred for nonemergent invasive coronary angiography (ICA). METHODS: CCTA data from individuals enrolled into the randomized controlled Computed Tomographic Angiography for Selective Cardiac Catheterization trial for an American College of Cardiology (ACC)/American Heart Association (AHA) guideline indication for ICA were analyzed. Site interpretation of CCTAs were compared to those analyzed by a cloud-based software (Cleerly, Inc.) that performs AI-QCT for stenosis determination, coronary vascular measurements and quantification and characterization of atherosclerotic plaque. CCTA interpretation and AI-QCT guided findings were related to MACE at 1-year follow-up. RESULTS: Seven hundred forty-seven stable patients (60 ± 12.2 years, 49% women) were included. Using AI-QCT, 9% of patients had no CAD compared with 34% for clinical CCTA interpretation. Application of AI-QCT to identify obstructive coronary stenosis at the ≥50% and ≥70% threshold would have reduced ICA by 87% and 95%, respectively. Clinical outcomes for patients without AI-QCT-identified obstructive stenosis was excellent; for 78% of patients with maximum stenosis < 50%, no cardiovascular death or acute myocardial infarction occurred. When applying an AI-QCT referral management approach to avoid ICA in patients with <50% or <70% stenosis, overall costs were reduced by 26% and 34%, respectively. CONCLUSIONS: In stable patients referred for ACC/AHA guideline-indicated nonemergent ICA, application of artificial intelligence and machine learning for AI-QCT can significantly reduce ICA rates and costs with no change in 1-year MACE.
APA Citation
Kim, Yumin; Choi, Andrew D.; Telluri, Anha; Lipkin, Isabella; Bradley, Andrew J.; Sidahmed, Alfateh; Jonas, Rebecca; Andreini, Daniele; Bathina, Ravi; Baggiano, Andrea; Cerci, Rodrigo; Choi, Eui-Young; Choi, Jung-Hyun; Choi, So-Yeon; Chung, Namsik; Cole, Jason; Doh, Joon-Hyung; Ha, Sang-Jin; Her, Ae-Young; Kepka, Cezary; Kim, Jang-Young; Kim, Jin Won; Kim, Sang-Wook; Kim, Woong; Pontone, Gianluca; Villines, Todd C.; Cho, Iksung; Danad, Ibrahim; Heo, Ran; Lee, Sang-Eun; Lee, Ji Hyun; and Park, Hyung-Bok, "Atherosclerosis Imaging Quantitative Computed Tomography (AI-QCT) to guide referral to invasive coronary angiography in the randomized controlled CONSERVE trial" (2023). GW Authored Works. Paper 2350.
https://hsrc.himmelfarb.gwu.edu/gwhpubs/2350
Department
Medicine
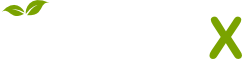
- Citations
- Citation Indexes: 12
- Usage
- Abstract Views: 13
- Captures
- Readers: 24
- Mentions
- News Mentions: 1