AI Evaluation of Stenosis on Coronary CT Angiography, Comparison With Quantitative Coronary Angiography and Fractional Flow Reserve: A CREDENCE Trial Substudy
Document Type
Journal Article
Publication Date
2-15-2022
Journal
JACC. Cardiovascular imaging
DOI
10.1016/j.jcmg.2021.10.020
Keywords
CCTA; artificial intelligence; atherosclerosis; coronary artery disease; coronary computed tomography; fractional flow reserve; quantitative coronary angiography
Abstract
OBJECTIVES: The study compared the performance for detection and grading of coronary stenoses using artificial intelligence-enabled quantitative coronary computed tomography angiography (AI-QCT) analyses to core lab-interpreted coronary computed tomography angiography (CTA), core lab quantitative coronary angiography (QCA), and invasive fractional flow reserve (FFR). BACKGROUND: Clinical reads of coronary CTA, especially by less experienced readers, may result in overestimation of coronary artery disease stenosis severity compared with expert interpretation. AI-based solutions applied to coronary CTA may overcome these limitations. METHODS: Coronary CTA, FFR, and QCA data from 303 stable patients (64 ± 10 years of age, 71% male) from the CREDENCE (Computed TomogRaphic Evaluation of Atherosclerotic DEtermiNants of Myocardial IsChEmia) trial were retrospectively analyzed using an Food and Drug Administration-cleared cloud-based software that performs AI-enabled coronary segmentation, lumen and vessel wall determination, plaque quantification and characterization, and stenosis determination. RESULTS: Disease prevalence was high, with 32.0%, 35.0%, 21.0%, and 13.0% demonstrating ≥50% stenosis in 0, 1, 2, and 3 coronary vessel territories, respectively. Average AI-QCT analysis time was 10.3 ± 2.7 minutes. AI-QCT evaluation demonstrated per-patient sensitivity, specificity, positive predictive value, negative predictive value, and accuracy of 94%, 68%, 81%, 90%, and 84%, respectively, for ≥50% stenosis, and of 94%, 82%, 69%, 97%, and 86%, respectively, for detection of ≥70% stenosis. There was high correlation between stenosis detected on AI-QCT evaluation vs QCA on a per-vessel and per-patient basis (intraclass correlation coefficient = 0.73 and 0.73, respectively; P < 0.001 for both). False positive AI-QCT findings were noted in in 62 of 848 (7.3%) vessels (stenosis of ≥70% by AI-QCT and QCA of <70%); however, 41 (66.1%) of these had an FFR of <0.8. CONCLUSIONS: A novel AI-based evaluation of coronary CTA enables rapid and accurate identification and exclusion of high-grade stenosis and with close agreement to blinded, core lab-interpreted quantitative coronary angiography. (Computed TomogRaphic Evaluation of Atherosclerotic DEtermiNants of Myocardial IsChEmia [CREDENCE]; NCT02173275).
APA Citation
Griffin, William F.; Choi, Andrew D.; Riess, Joanna S.; Marques, Hugo; Chang, Hyuk-Jae; Choi, Jung Hyun; Doh, Joon-Hyung; Her, Ae-Young; Koo, Bon-Kwon; Nam, Chang-Wook; Park, Hyung-Bok; Shin, Sang-Hoon; Cole, Jason; Gimelli, Alessia; Khan, Muhammad Akram; Lu, Bin; Gao, Yang; Nabi, Faisal; Nakazato, Ryo; Schoepf, U Joseph; Driessen, Roel S.; Bom, Michiel J.; Thompson, Randall; Jang, James J.; Ridner, Michael; Rowan, Chris; Avelar, Erick; Généreux, Philippe; Knaapen, Paul; de Waard, Guus A.; Pontone, Gianluca; and Andreini, Daniele, "AI Evaluation of Stenosis on Coronary CT Angiography, Comparison With Quantitative Coronary Angiography and Fractional Flow Reserve: A CREDENCE Trial Substudy" (2022). GW Authored Works. Paper 151.
https://hsrc.himmelfarb.gwu.edu/gwhpubs/151
Department
Radiology
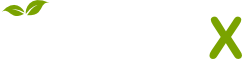
- Citations
- Citation Indexes: 95
- Usage
- Abstract Views: 30
- Captures
- Readers: 106
- Mentions
- Blog Mentions: 1
- News Mentions: 2