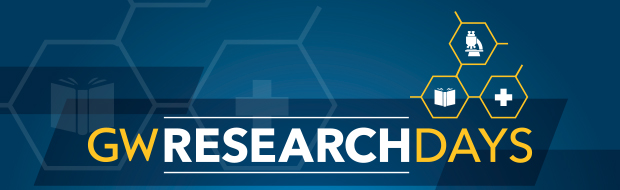
School of Medicine and Health Sciences Poster Presentations
Document Type
Poster
Status
Medical Student
Abstract Category
Cancer/Oncology
Keywords
proteomics, cancer, mislabeling, data
Publication Date
Spring 2019
Abstract
Sample mislabeling is a pervasive problem in biomedical research, especially large-scale multi-omics studies, contributing to errors and leading to false conclusions. The Food and Drug Administration (FDA) and the National Cancer Institute Clinical Proteomic Tumor Analysis Consortium (NCI-CPTA) have launched a data science challenge to address this problem. We developed a novel machine learning based approach that combines traditional machine learning with learning from cancer genomics literature to identify mislabeled tumors in the NCI-CPTA Multi-omics Mislabeling Challenge.
The training data contained a sample of a tumor from 80 different patients, each containing features on gender, microsatellite instability (MSI) status, and proteomics data for up to 4119 proteins. Competition organizers systematically mislabeled 10% of the data, which lead to incorrect gender or MSI status, relative to proteomics data, for most mislabeled samples.
To create a model to identify mislabeled samples, we used proteomics data to predict both the correct gender and MSI and compare predictions to the the given data. This would identify mislabeled instances of sample swapping and, potentially, duplication and shifting as well. Gender mislabeling was predicted using genes unique to the y chromosome and associated with cancer. We turned these genes into dummy variables (present/not present) and evaluated each protein’s predictive value using kappa statistics. Two genes far out-performed the rest, DDX3Y and RPS4Y1, which together gave us the gender prediction in our test set. MSI was predicting by applying dimensionality reduction and a logistic regression classifier. First, we conducted an F-test with an adjusted p-threshold of 0.05 to identify 31 proteins that are dysregulated in unstable tumor genomes (high MSI) compared to stable tumor genomes (low MSI). In addition, 38 proteins in our dataset were identified in medical literature to be associated with MSI and were included in our dataset. We used these 62 proteins in a logistic regression model to predict MSI.
When comparing the prediction of gender and MSI by our model to the actual prediction, any mismatches were therefore invalid. Results on the unseen test set yielded a sensitivity of 0.83 and a specificity of 0.50.
Creative Commons License
This work is licensed under a Creative Commons Attribution-Noncommercial-No Derivative Works 4.0 License.
Open Access
1
Included in
NCI Multi-omics Mislabeling Challenge: A Machine Learning Approach
Sample mislabeling is a pervasive problem in biomedical research, especially large-scale multi-omics studies, contributing to errors and leading to false conclusions. The Food and Drug Administration (FDA) and the National Cancer Institute Clinical Proteomic Tumor Analysis Consortium (NCI-CPTA) have launched a data science challenge to address this problem. We developed a novel machine learning based approach that combines traditional machine learning with learning from cancer genomics literature to identify mislabeled tumors in the NCI-CPTA Multi-omics Mislabeling Challenge.
The training data contained a sample of a tumor from 80 different patients, each containing features on gender, microsatellite instability (MSI) status, and proteomics data for up to 4119 proteins. Competition organizers systematically mislabeled 10% of the data, which lead to incorrect gender or MSI status, relative to proteomics data, for most mislabeled samples.
To create a model to identify mislabeled samples, we used proteomics data to predict both the correct gender and MSI and compare predictions to the the given data. This would identify mislabeled instances of sample swapping and, potentially, duplication and shifting as well. Gender mislabeling was predicted using genes unique to the y chromosome and associated with cancer. We turned these genes into dummy variables (present/not present) and evaluated each protein’s predictive value using kappa statistics. Two genes far out-performed the rest, DDX3Y and RPS4Y1, which together gave us the gender prediction in our test set. MSI was predicting by applying dimensionality reduction and a logistic regression classifier. First, we conducted an F-test with an adjusted p-threshold of 0.05 to identify 31 proteins that are dysregulated in unstable tumor genomes (high MSI) compared to stable tumor genomes (low MSI). In addition, 38 proteins in our dataset were identified in medical literature to be associated with MSI and were included in our dataset. We used these 62 proteins in a logistic regression model to predict MSI.
When comparing the prediction of gender and MSI by our model to the actual prediction, any mismatches were therefore invalid. Results on the unseen test set yielded a sensitivity of 0.83 and a specificity of 0.50.
Comments
Presented at Research Days 2019.