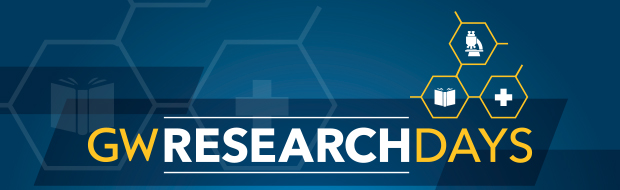
School of Medicine and Health Sciences Poster Presentations
A Machine Learning Approach to Mapping Co-regulated Variant Loci and Gene Expression over Time
Document Type
Poster
Abstract Category
Basic Biomedical Sciences
Keywords
bioinformatics, statistics, cancer
Publication Date
Spring 5-1-2019
Abstract
We developed a machine learning analysis pipeline to discover functional gene variants by examining the effect of RNA containing single nucleotide variants (SNVs) on gene expression at cis- and trans- genomic locations over time. This reflects a hypothesis of genetic co-regulation where, as the relative presence of a particular variant allele seen in RNA transcription changes over time (due to changing cellular requirements), gene expression elsewhere in the genome is affected as a result . We believe this analysis pipeline can give novel mechanistic insights into a wide range of basic and translational cell biology questions, particularly on the evolution of drug resistance in cancer cells. In order to measure changing cellular requirements in cancer cell lines, we conducted paired-end RNA sequencing on human melanoma cell line WM164 under 4 experimental conditions: with and without histone deacytelase (HDAC) inhibition, and with and without IFN-gamma treatment. This was done over 8 time points each, for a total of 32 samples. We then aligned the RNA-sequencing reads to the human genome and called variants. Our pipeline starts with clustering in Graphia Professional, which uses a graph-based approach to build relationships between genes. Each VAF or gene expression “time-course” groups with another if it meets a minimum Pearson correlation of 0.96. As a result, genes with similar expression trends and VAF trends are connected to each other in a structured graph. We then used a machine learning method called the Markov Cluster algorithm (MCL) to partition the graph into formal clusters by looking for packs of highly interconnected genes. We then built custom R modules to scan through the clusters to find pairs of VAF and gene expression profiles that cluster together in two samples or more. Once found, each pair was categorized as a cis- relationship if they were less than one million base-pairs apart, and trans- otherwise. All 60,963 VAF and gene expression profiles were clustered into 3,730 clusters, where each cluster represents a certain pattern of RNA regulation through time. Using custom R scripts, we discovered 440 co-regulated VAF containing positions and gene expression profiles. Further work will include Protein-Protein Interaction (PPI) analyses to validate findings, especially in a larger data set.
Open Access
1
A Machine Learning Approach to Mapping Co-regulated Variant Loci and Gene Expression over Time
We developed a machine learning analysis pipeline to discover functional gene variants by examining the effect of RNA containing single nucleotide variants (SNVs) on gene expression at cis- and trans- genomic locations over time. This reflects a hypothesis of genetic co-regulation where, as the relative presence of a particular variant allele seen in RNA transcription changes over time (due to changing cellular requirements), gene expression elsewhere in the genome is affected as a result . We believe this analysis pipeline can give novel mechanistic insights into a wide range of basic and translational cell biology questions, particularly on the evolution of drug resistance in cancer cells. In order to measure changing cellular requirements in cancer cell lines, we conducted paired-end RNA sequencing on human melanoma cell line WM164 under 4 experimental conditions: with and without histone deacytelase (HDAC) inhibition, and with and without IFN-gamma treatment. This was done over 8 time points each, for a total of 32 samples. We then aligned the RNA-sequencing reads to the human genome and called variants. Our pipeline starts with clustering in Graphia Professional, which uses a graph-based approach to build relationships between genes. Each VAF or gene expression “time-course” groups with another if it meets a minimum Pearson correlation of 0.96. As a result, genes with similar expression trends and VAF trends are connected to each other in a structured graph. We then used a machine learning method called the Markov Cluster algorithm (MCL) to partition the graph into formal clusters by looking for packs of highly interconnected genes. We then built custom R modules to scan through the clusters to find pairs of VAF and gene expression profiles that cluster together in two samples or more. Once found, each pair was categorized as a cis- relationship if they were less than one million base-pairs apart, and trans- otherwise. All 60,963 VAF and gene expression profiles were clustered into 3,730 clusters, where each cluster represents a certain pattern of RNA regulation through time. Using custom R scripts, we discovered 440 co-regulated VAF containing positions and gene expression profiles. Further work will include Protein-Protein Interaction (PPI) analyses to validate findings, especially in a larger data set.
Comments
Presented at Research Days 2019.