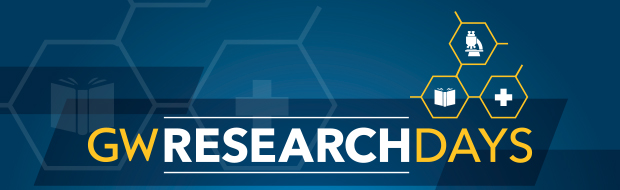
Children's National Health System Posters
The relationship of language and explicit memory in pediatric epilepsy
Poster Number
354
Document Type
Poster
Status
Staff
Abstract Category
Neuroscience
Keywords
Children, epilepsy, neuropsychology, language, memory
Publication Date
Spring 2018
Abstract
Objective: Memory and language are often at risk in pediatric epilepsy but findings are mixed. We investigated the relationship between explicit memory and language in children with epilepsy and controls using data-driven statistical methods applying model-based clustering to explore if different cognitive profiles emerge.
Participants and Methods: 276 children ages 6.1 to 17.5 were evaluated as part of ongoing studies. 149 patients with focal epilepsy (EPI) and 127 typically developing children (TD) completed language (EOWPVT, WASI VIQ) and memory (CVLT-C) measures. Six normative z-scores were used (4 from CVLT-C) for: (1) Pearson r correlations and (2) the model-based clustering procedure. For the latter, we applied Gaussian finite mixture model-based clustering on the 6 scores through R package mclust using Bayes Information Criterion to determine if scores clustered into distinct profiles and how EPI and TD fell in each profile. Epilepsy variables were compared to ascertain if they related to group membership.
Results: Language scores were positively correlated with all memory scores (r’s>0.26, p’s ≤ 0.001). The optimal cluster number was 2 across TD and EPI; language and memory separated into high (n=206, M z= 0.44, EPI=46%, TD=54%) and low performance groups (n=70, M z=-1.04, EPI=77%, TD=23%). The groups did not differ on age. Within EPI only, 2 was also the optimal number of clusters. Even though the majority of the low group was EPI, it represented only 1/3 of all patients. Epilepsy variables were no different across groups.
Conclusions: Language and memory are associated and when included in model-based clustering two distinct groups were revealed. The method found heterogeneity within EPI that may explain prior mixed results. Parsing groups in a data driven manner may reveal subgroups that may have biomarkers for their cognitive profiles thus indicating a protective factor or a risk. Further studies will test for biomarkers (e.g. findings from structural or functional imaging) that predict these cognitive profiles.
Creative Commons License
This work is licensed under a Creative Commons Attribution-Noncommercial-No Derivative Works 4.0 License.
Open Access
1
The relationship of language and explicit memory in pediatric epilepsy
Objective: Memory and language are often at risk in pediatric epilepsy but findings are mixed. We investigated the relationship between explicit memory and language in children with epilepsy and controls using data-driven statistical methods applying model-based clustering to explore if different cognitive profiles emerge.
Participants and Methods: 276 children ages 6.1 to 17.5 were evaluated as part of ongoing studies. 149 patients with focal epilepsy (EPI) and 127 typically developing children (TD) completed language (EOWPVT, WASI VIQ) and memory (CVLT-C) measures. Six normative z-scores were used (4 from CVLT-C) for: (1) Pearson r correlations and (2) the model-based clustering procedure. For the latter, we applied Gaussian finite mixture model-based clustering on the 6 scores through R package mclust using Bayes Information Criterion to determine if scores clustered into distinct profiles and how EPI and TD fell in each profile. Epilepsy variables were compared to ascertain if they related to group membership.
Results: Language scores were positively correlated with all memory scores (r’s>0.26, p’s ≤ 0.001). The optimal cluster number was 2 across TD and EPI; language and memory separated into high (n=206, M z= 0.44, EPI=46%, TD=54%) and low performance groups (n=70, M z=-1.04, EPI=77%, TD=23%). The groups did not differ on age. Within EPI only, 2 was also the optimal number of clusters. Even though the majority of the low group was EPI, it represented only 1/3 of all patients. Epilepsy variables were no different across groups.
Conclusions: Language and memory are associated and when included in model-based clustering two distinct groups were revealed. The method found heterogeneity within EPI that may explain prior mixed results. Parsing groups in a data driven manner may reveal subgroups that may have biomarkers for their cognitive profiles thus indicating a protective factor or a risk. Further studies will test for biomarkers (e.g. findings from structural or functional imaging) that predict these cognitive profiles.