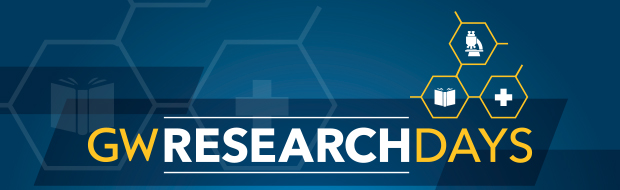
Department of Biomedical Engineering Posters and Presentations
Motion Learning For Emotional Interaction And Imitation Of Children With Autism Spectrum Disorder
Document Type
Poster
Keywords
autism, robotics, imitation, behavior therapy
Publication Date
4-2017
Abstract
Children with autism spectrum disorder (ASD) often display difficulty with social interaction. We aim to improve their emotional communication skills through the use of an assistive robotic framework, which will identify and dynamically respond to emotion-revealing body language detected through multimodal inputs.
Children with ASD may differ from neurotypical children in areas such as such as sensory interpretation, communication methods, and emotional response. As a result, children with autism may have difficulty interacting with their peers. Studies show that the incorporation of robots, music, and imitation techniques into therapy sessions all promote the child’s level of interest in interacting with others.
We propose the use of an autonomous social robot to identify emotional movements, and to reciprocate them through imitation, in order to form empathy with the child and encourage engagement. This will be accomplished by using multi-dimensional motion learning of dynamic movement primitives.
A dynamic movement primitive (DMP) is a generalized task with specific position goals and end points joined in a sequence to create a scalable movement. Robots utilize DMPs to reproduce core movements in variable settings.
For standard DMP techniques, such as DMP with weighted least squares (WLS), the duration, position, and intensity of the activation weights are preset. Usually, they are evenly distributed across the duration of the movement. For DMP with GMR, the span and placement of the activation functions are modified as the motion is learned.
DMP with GMR is a better method for computer replication of human movements (motion learning) than traditional DMP methods due to its capability for producing more accurate simulations while using the same number of activation states. Our current work can successfully detect the user, generate a skeletal framework, and track, record, and replicate movements in a 1D trajectory. We can record and generate 3D representations of the user’s movements, and are working towards 3D replication and testing next.
Creative Commons License
This work is licensed under a Creative Commons Attribution-Noncommercial-No Derivative Works 4.0 License.
Open Access
1
Motion Learning For Emotional Interaction And Imitation Of Children With Autism Spectrum Disorder
Children with autism spectrum disorder (ASD) often display difficulty with social interaction. We aim to improve their emotional communication skills through the use of an assistive robotic framework, which will identify and dynamically respond to emotion-revealing body language detected through multimodal inputs.
Children with ASD may differ from neurotypical children in areas such as such as sensory interpretation, communication methods, and emotional response. As a result, children with autism may have difficulty interacting with their peers. Studies show that the incorporation of robots, music, and imitation techniques into therapy sessions all promote the child’s level of interest in interacting with others.
We propose the use of an autonomous social robot to identify emotional movements, and to reciprocate them through imitation, in order to form empathy with the child and encourage engagement. This will be accomplished by using multi-dimensional motion learning of dynamic movement primitives.
A dynamic movement primitive (DMP) is a generalized task with specific position goals and end points joined in a sequence to create a scalable movement. Robots utilize DMPs to reproduce core movements in variable settings.
For standard DMP techniques, such as DMP with weighted least squares (WLS), the duration, position, and intensity of the activation weights are preset. Usually, they are evenly distributed across the duration of the movement. For DMP with GMR, the span and placement of the activation functions are modified as the motion is learned.
DMP with GMR is a better method for computer replication of human movements (motion learning) than traditional DMP methods due to its capability for producing more accurate simulations while using the same number of activation states. Our current work can successfully detect the user, generate a skeletal framework, and track, record, and replicate movements in a 1D trajectory. We can record and generate 3D representations of the user’s movements, and are working towards 3D replication and testing next.
Comments
To be presented at GW Annual Research Days 2017.