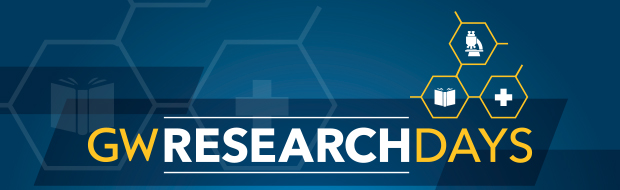
Milken Institute School of Public Health Poster Presentations (Marvin Center & Video)
A 3D Method for Human Body Modeling and Composition Assessment
Poster Number
97
Document Type
Poster
Publication Date
3-2016
Abstract
Excess body fat is a key underlying factor in the development of numerous chronic diseases, including type II diabetes, heart disease, stroke, and cancer. The primary objective of this project is to develop an accurate, reliable, convenient and cost-effective method of scanning body surface shapes and to use this technique to accurately assess the percent body fat (%BF). This technique will have the potential to be implemented in clinical or personal settings and utilized as a public health research tool. For this project, we are developing a highly innovative approach for non-rigid registration which will make it possible to capture human shapes using one commodity depth camera (Microsoft Kinect®). A supervised Machine Learning Algorithm is then used to map the resulted 3D body representation to accurate %BF. The use of Machine Learning techniques in calculating %BF is a significant innovation and will be a major improvement on the currently used methods such as Siri or Brozek equations that predict %BF based on density and cohort.
Creative Commons License
This work is licensed under a Creative Commons Attribution-Noncommercial-No Derivative Works 4.0 License.
Open Access
1
A 3D Method for Human Body Modeling and Composition Assessment
Excess body fat is a key underlying factor in the development of numerous chronic diseases, including type II diabetes, heart disease, stroke, and cancer. The primary objective of this project is to develop an accurate, reliable, convenient and cost-effective method of scanning body surface shapes and to use this technique to accurately assess the percent body fat (%BF). This technique will have the potential to be implemented in clinical or personal settings and utilized as a public health research tool. For this project, we are developing a highly innovative approach for non-rigid registration which will make it possible to capture human shapes using one commodity depth camera (Microsoft Kinect®). A supervised Machine Learning Algorithm is then used to map the resulted 3D body representation to accurate %BF. The use of Machine Learning techniques in calculating %BF is a significant innovation and will be a major improvement on the currently used methods such as Siri or Brozek equations that predict %BF based on density and cohort.
Comments
Presented at: GW Research Days 2016