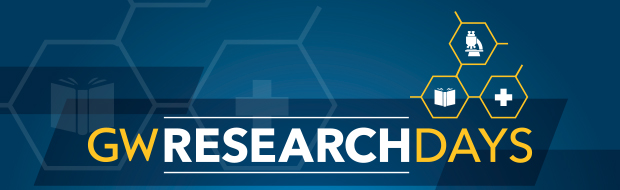
School of Medicine and Health Sciences Poster Presentations
Natural Language Inputs for Medication Reconciliation: A Feasibility Study
Poster Number
301
Document Type
Poster
Publication Date
3-2016
Abstract
Introduction: Medication errors and adverse events are common throughout the health care continuum, often at transitions of care. The clinical and financial impact of these events is significant, both to the patient and the healthcare system. In 2005, The Joint Commission identified medication reconciliation as National Patient Safety Goal No. 8 in effort to reduce errors and subsequently improve patient outcomes. Our study aims to address medication reconciliation using an innovative approach that leverages a mobile natural language processing (NLP) software application for vocal medication intake. In this study we captured recordings of medications spoken in a variety of dialects from laypersons in order to determine the accuracy and sensitivity of voice recognition with natural language processing in various environmental settings.
Methods: The study protocol was approved by the IRB. We recruited and interviewed adult volunteers who spoke English on the National Mall in Washington, DC. Participants completed a demographics survey and were asked to recall up to five medications, supplements, or vitamins of their choosing to speak into the IOS mobile application run on an iPhone. The application processed the input by recognizing the speech from the voice recognition engine and then matching recognized medications with a grammar derived from a limited National Drug Code (NDC) directory. If identifiable, the screen displayed text listing the items spoken.
Results: Forty-seven participants were recruited and forty-six inputs were suitable for analysis. Participants represented four countries and seventeen US states.
We used industry standard measures for speech recognition Word Error Rate (WER) and information retrieval measures of Precision and Recall in order to gauge the accuracy of the application at medication recognition. Without accounting for medications spoken that were not listed in the NDC directory, the application had a Recall rate of 97% with Precision of 77% and WER of 29%. Including only identifiable medications, Recall remained 97% and Precision increased to 82%.
Conclusions: The results of this feasibility study suggest that the use of voice recognition and NLP for medication intake can be effective. We found that even with a variety of layperson inputs recorded against a wide range of background noise levels, the NLP software accurately detected medication inputs from laypersons with high precision and recall rates. In future testing, we plan to include more common names of medications to improve recognition. We also want to control ambient noise by testing in task-appropriate environments, including the home, clinic or hospital.
Creative Commons License
This work is licensed under a Creative Commons Attribution-Noncommercial-No Derivative Works 4.0 License.
Open Access
1
Natural Language Inputs for Medication Reconciliation: A Feasibility Study
Introduction: Medication errors and adverse events are common throughout the health care continuum, often at transitions of care. The clinical and financial impact of these events is significant, both to the patient and the healthcare system. In 2005, The Joint Commission identified medication reconciliation as National Patient Safety Goal No. 8 in effort to reduce errors and subsequently improve patient outcomes. Our study aims to address medication reconciliation using an innovative approach that leverages a mobile natural language processing (NLP) software application for vocal medication intake. In this study we captured recordings of medications spoken in a variety of dialects from laypersons in order to determine the accuracy and sensitivity of voice recognition with natural language processing in various environmental settings.
Methods: The study protocol was approved by the IRB. We recruited and interviewed adult volunteers who spoke English on the National Mall in Washington, DC. Participants completed a demographics survey and were asked to recall up to five medications, supplements, or vitamins of their choosing to speak into the IOS mobile application run on an iPhone. The application processed the input by recognizing the speech from the voice recognition engine and then matching recognized medications with a grammar derived from a limited National Drug Code (NDC) directory. If identifiable, the screen displayed text listing the items spoken.
Results: Forty-seven participants were recruited and forty-six inputs were suitable for analysis. Participants represented four countries and seventeen US states.
We used industry standard measures for speech recognition Word Error Rate (WER) and information retrieval measures of Precision and Recall in order to gauge the accuracy of the application at medication recognition. Without accounting for medications spoken that were not listed in the NDC directory, the application had a Recall rate of 97% with Precision of 77% and WER of 29%. Including only identifiable medications, Recall remained 97% and Precision increased to 82%.
Conclusions: The results of this feasibility study suggest that the use of voice recognition and NLP for medication intake can be effective. We found that even with a variety of layperson inputs recorded against a wide range of background noise levels, the NLP software accurately detected medication inputs from laypersons with high precision and recall rates. In future testing, we plan to include more common names of medications to improve recognition. We also want to control ambient noise by testing in task-appropriate environments, including the home, clinic or hospital.
Comments
Presented at: GW Research Days 2016