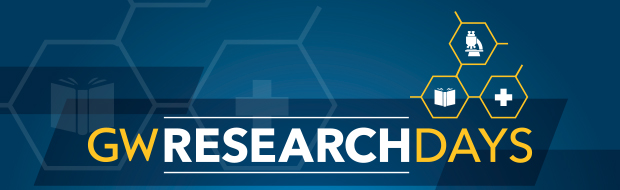
Milken Institute School of Public Health Poster Presentations (Marvin Center & Video)
Using the Inverse Maximum Ratio-Λ as a Technique to Quantify Surface Uniformity
Poster Number
48
Document Type
Poster
Publication Date
3-2016
Abstract
Background
Techniques used in data variability assessment are subsequently used to draw conclusions regarding the “spread”/uniformity of data curves. Due to the limitations of these techniques, they are not adequate for circumstances where data manifest with multiple peaks (bimodal, multi-modal). As such manifestations of data are common in various fields, a sound mathematical approach to quantify the uniformity of such data could prove to be useful in a number of different ways. Extrapolating such an approach to quantify the uniformity of surfaces in 3-dimensional space could further expand its utility.
Methods
We proposed and validated a new mathematical metric, the Inverse Maximum Ratio, to quantify the uniformity behavior of bimodal and multi-modal data in 2 dimensions. Firstly, a universal mathematical definition of the Inverse Maximum Ratio (Λ) was derived and its expected value and variance were examined under various conditions. The performance of Λ was then assesed under various uni-modal, bimodal and multimodal data conditions, and the finite bounds of the metric were examined. The utility of Λ in quantifying the uniformity of surfaces in 3-dimensional space was then examined using several simulated surfaces with various ‘non-uniformity’ manifestations.
Results
In both 2 and 3-dimensions, Λ performed consistently across all tested conditions. The range of the metric was determined to be within [0, 1] and estimates closer to the upper bound indicated more ‘uniform’ presentations of data. From derivations of its expected value and variance as well as from graphical assessments, it’s performance was determined to be distribution independent and reliabile across various manifestations of data (unimodal, bimodal, multi-modal in 2 dimensional conditions). Λ was also determined to be superior to commonly used variability assessment techniques in capturing the uniformity of data curves. Further, Λ was also determined to be equivalently useful in 3-dimensional space to quantify the uniformity of various surfaces. The bounds, behavior and consistency of the metric was identical in 2 and 3-dimensional conditions.
Conclusion
We believe that the proposed metric not only performs consistently across all presentations of data in both 2 and 3-dimentions, but also that it outperforms the standard techniques in bimodal and multi-modal presentations of data. This metric’s consistent performance in all tested conditions make it a reliable tool for use in fields such as biomechanics, immunology, material sciences etc. where such presentations of data are common.
Creative Commons License
This work is licensed under a Creative Commons Attribution-Noncommercial-No Derivative Works 4.0 License.
Open Access
1
Using the Inverse Maximum Ratio-Λ as a Technique to Quantify Surface Uniformity
Background
Techniques used in data variability assessment are subsequently used to draw conclusions regarding the “spread”/uniformity of data curves. Due to the limitations of these techniques, they are not adequate for circumstances where data manifest with multiple peaks (bimodal, multi-modal). As such manifestations of data are common in various fields, a sound mathematical approach to quantify the uniformity of such data could prove to be useful in a number of different ways. Extrapolating such an approach to quantify the uniformity of surfaces in 3-dimensional space could further expand its utility.
Methods
We proposed and validated a new mathematical metric, the Inverse Maximum Ratio, to quantify the uniformity behavior of bimodal and multi-modal data in 2 dimensions. Firstly, a universal mathematical definition of the Inverse Maximum Ratio (Λ) was derived and its expected value and variance were examined under various conditions. The performance of Λ was then assesed under various uni-modal, bimodal and multimodal data conditions, and the finite bounds of the metric were examined. The utility of Λ in quantifying the uniformity of surfaces in 3-dimensional space was then examined using several simulated surfaces with various ‘non-uniformity’ manifestations.
Results
In both 2 and 3-dimensions, Λ performed consistently across all tested conditions. The range of the metric was determined to be within [0, 1] and estimates closer to the upper bound indicated more ‘uniform’ presentations of data. From derivations of its expected value and variance as well as from graphical assessments, it’s performance was determined to be distribution independent and reliabile across various manifestations of data (unimodal, bimodal, multi-modal in 2 dimensional conditions). Λ was also determined to be superior to commonly used variability assessment techniques in capturing the uniformity of data curves. Further, Λ was also determined to be equivalently useful in 3-dimensional space to quantify the uniformity of various surfaces. The bounds, behavior and consistency of the metric was identical in 2 and 3-dimensional conditions.
Conclusion
We believe that the proposed metric not only performs consistently across all presentations of data in both 2 and 3-dimentions, but also that it outperforms the standard techniques in bimodal and multi-modal presentations of data. This metric’s consistent performance in all tested conditions make it a reliable tool for use in fields such as biomechanics, immunology, material sciences etc. where such presentations of data are common.
Comments
Presented at: GW Research Days 2016