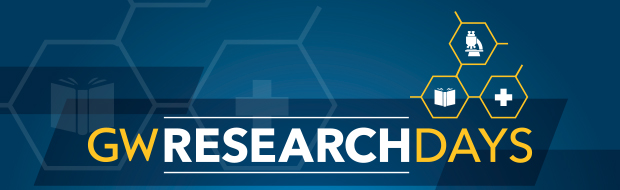
Department of Biomedical Engineering Posters and Presentations
Detecting Anomalous in Medical Data Using Hierarchical Temporal Memory
Poster Number
112
Document Type
Poster
Publication Date
3-2016
Abstract
Detecting anomalous in medical field has always been an important issue, for correctly recognizing anomalous from the data such as echocardiogram images and ECG signals can help the doctor take the right action when time is limited. However , conventional methods show limited ability in both detecting anomalous accurately and in real time .This paper use a new data mining technique – Hierarchical Temporal Memory. Aiming to model the learning process of Neocortex ,HTM learns both the spatial and temporal pattern of the data , thus can analysis and detect anomalous in real time and adaptively as the data changes . Secondly , HTM uses a hierarchical learning structure , which is able to capture the spatial and temporal pattern of the data in a large scale with practical amount of memory . This research apply HTM to the medical data of echocardiogram images and ECG signals , the result proves that HTM is more powerful than prvious methods in detecting and even predicting the data pattern in real time.
Creative Commons License
This work is licensed under a Creative Commons Attribution-Noncommercial-No Derivative Works 4.0 License.
Open Access
1
Detecting Anomalous in Medical Data Using Hierarchical Temporal Memory
Detecting anomalous in medical field has always been an important issue, for correctly recognizing anomalous from the data such as echocardiogram images and ECG signals can help the doctor take the right action when time is limited. However , conventional methods show limited ability in both detecting anomalous accurately and in real time .This paper use a new data mining technique – Hierarchical Temporal Memory. Aiming to model the learning process of Neocortex ,HTM learns both the spatial and temporal pattern of the data , thus can analysis and detect anomalous in real time and adaptively as the data changes . Secondly , HTM uses a hierarchical learning structure , which is able to capture the spatial and temporal pattern of the data in a large scale with practical amount of memory . This research apply HTM to the medical data of echocardiogram images and ECG signals , the result proves that HTM is more powerful than prvious methods in detecting and even predicting the data pattern in real time.
Comments
Presented at: GW Research Days 2016